Client Background + Problem
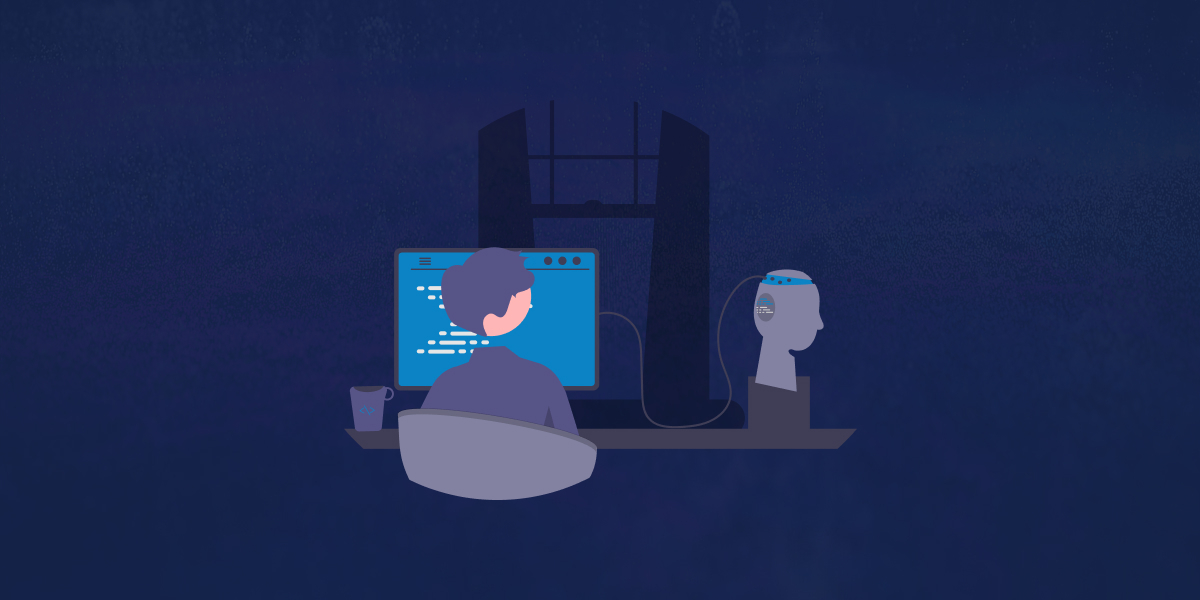
In the competitive world of healthcare education, where medical professionals are required to enhance their knowledge and skills to keep licenses current, effective advertising plays a pivotal role. Most professionals have to look for the latest courses and content for specialty areas of medical practice. Not every provider will have this content or the ability to report progress on it to licensing boards.
This case study focuses on a client operating in the healthcare education space, selling continuing education courses to nurses, physicians, dentists, and other medical professionals.
Over a four-month period from January of 2023 to April 2023, the client experienced a remarkable increase in revenue, with a notable improvement to return on ad spend.
In this blog post, we will delve into the optimizations implemented that contributed to success in a challenging landscape.
- The client was facing a problem with Non-Brand campaigns having a declining return-on-ad-spend (ROAS) year-over-year (YoY), for the last three years.
- The account was using a sophisticated campaign architecture with manual bidding algorithms that was highly dependent on ad position based metrics.
- With the recent changes to the platform over the last 18 months, it was time to reconsider if the strategy used in the past (which was very profitable for almost 10 years) had run its course.
- The recent AI/ML changes in Google Ads over the last two years.
- Moving to a campaign and conversion architecture which aggregates and feeds the most and best data points to Google’s machine learning
- Our role as the account manager moving away from sophisticated campaign management (largely measured by number of changes in account), to quality data stewardship and AI/ML
We told them we believed a critical mass had been reached with Google Ads ML maturity in the last two years, and that it was time to go all in on letting the platform optimize for results with oversight and guidance. Here's what the response to the pitch was:
- The client bought into the idea starting with a pilot test.
- The pilot test was wildly successful.
- We transitioned one profession at a time to the new architecture ensuring results along the way.
Over a four-month period from January to April 2023, the client experienced significant revenue growth, with a notable improvement to return on ad spend.
During the period of January 1 to April 26, 2023, the client witnessed exceptional results when compared to the same period in 2021*. The figures speak for themselves:
- AdSpend: $260,193.36 (-6.08%)
- Revenue: $2,029,910.35 (+28.06%, +$444,742.66)
- Gross Margin: 87% (+5.65%)
- Return on Ad Spend (ROAS): 780% (+36.35%)
*Client chooses to compare odd years to odd years due to medical license expiration schedules: 2023 vs. 2021
In this blog post, we will delve into some of the optimizations implemented that contributed to success.
Google Ads AI / ML Optimization #1: Restructuring the Account
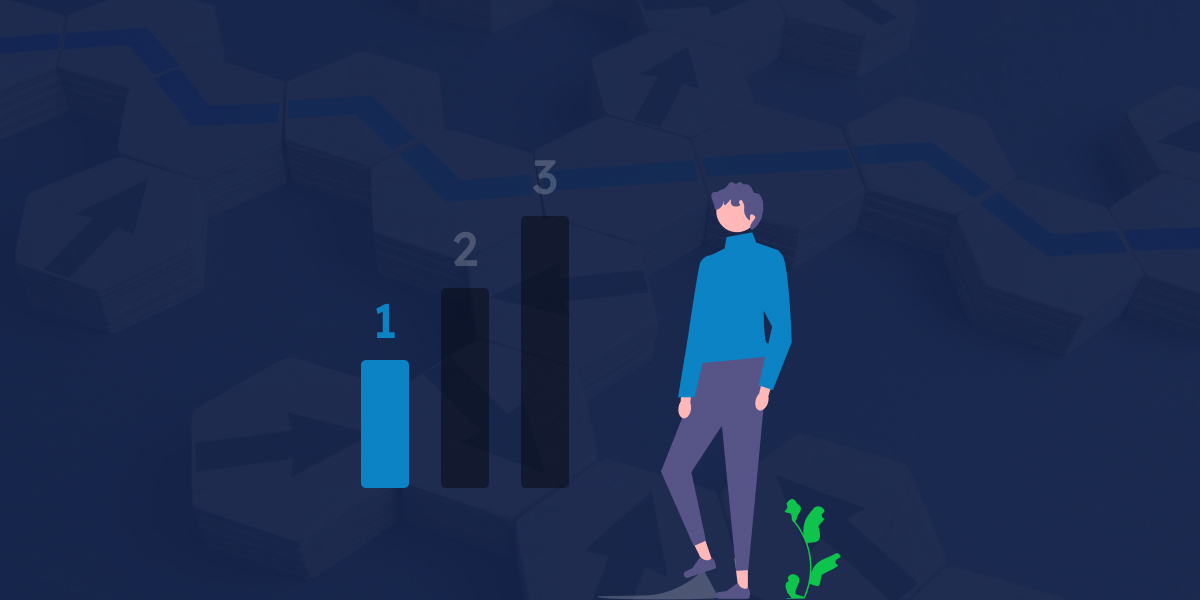
To leverage the power of machine learning, a comprehensive restructuring of the client's advertising account was necessary.
In today’s landscape, you need to fine tune and train Google’s machine learning to provide top tier returns
The following steps were taken to restructure the account to effectively use machine learning:
- Bid Strategy: using the best and most appropriate automated bid strategy at the campaign level for the purpose of the campaign.
- Ad Group Features: Setting Target Return on Ad Spend (tROAS) targets at the ad group level for high-value campaigns to feed Google’s machine learning with the ROAS we are trying to achieve.
- Ad Group Organization: organizing ad groups from keywords to concepts in order to give the platform more aggregated conversion data at the ad group level.
- Audience Targeting Options: We leveraged advanced targeting options to refine the audience attributes that the platform could use to enhance campaign targeting & performance.
The restructuring process resulted in improved ad spend optimization and significantly enhanced ad performance, laying a strong foundation for future success.
Google Ads AI / ML Optimization #2: Consolidating Device Types Into One Campaign
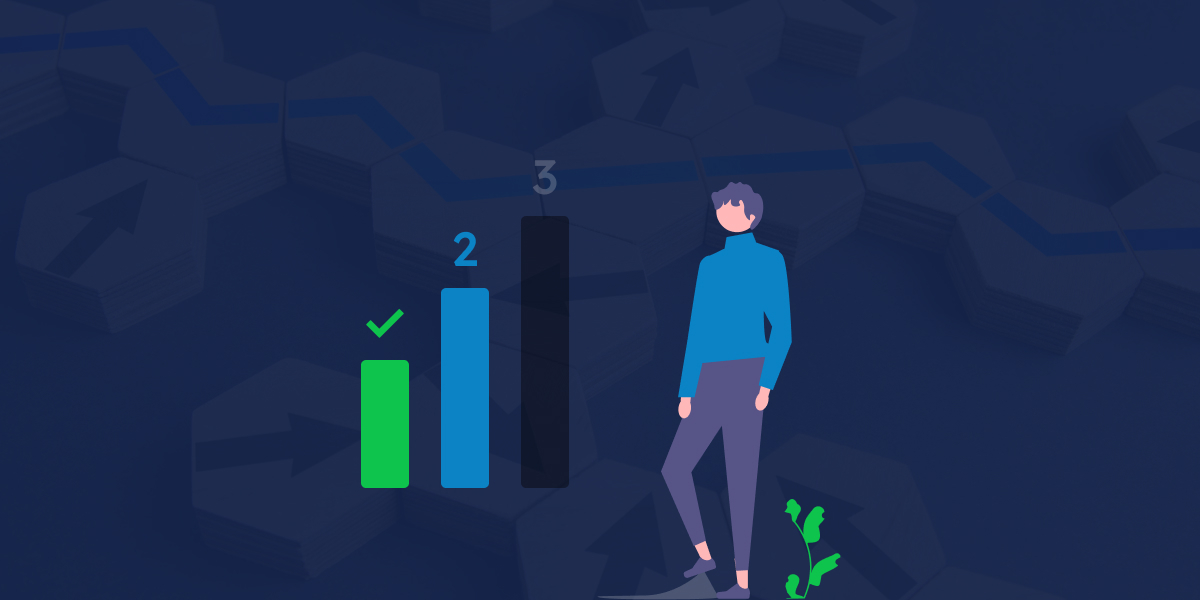
Consolidating device types and or match types into a single campaign proved to be a pivotal step in achieving greater success for our client.
The steps involved in this process were as follows:
- Evaluation of Device Performance: We analyzed the performance metrics of different device types (mobile, desktop, tablet) to identify patterns and insights for keywords and audiences.
- Aggregation of Campaigns: Using the data-driven insights gained, we aggregated campaign targeting to all device types with the best keywords and audiences, ensuring an optimal reach.
The consolidation of device types allowed for more aggregation of conversion volume and audience data points, which fueled the machine learning features in use, resulting in increased ad reach for the best audiences who were most likely to convert.
Google Ads AI/ML Optimization #3: Turned On Automated Bidding & Targeting
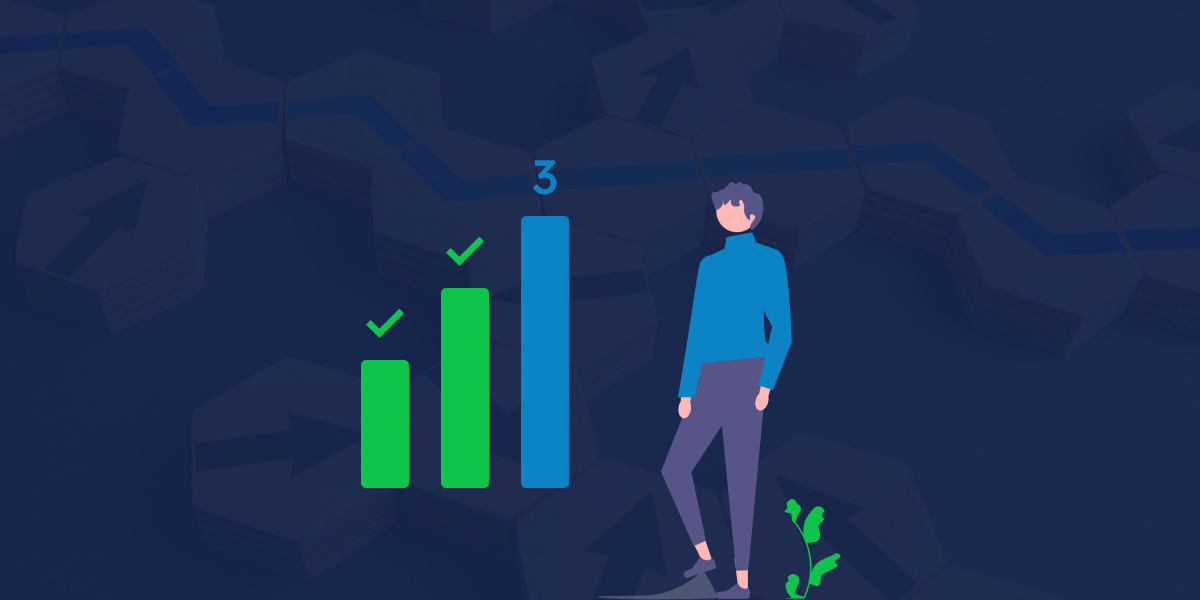
Once we restructured the account, made optimizations to the keywords and devices, and set Google up to use as many data points as possible, we were able to turn on Machine Learning. To drive the most optimal performance for non-brand campaigns, we adopted tROAS bid strategies, which proved to be highly effective.
How Do tROAS Bid Strategies work?
- Google's machine learning algorithms analyze historical data and real-time signals to adjust bids and allocate budgets to maximize the chances of achieving a specified target ROAS.
- This bid strategy is particularly useful for non-brand campaigns, where the focus is on driving revenue and measuring the effectiveness of advertising investments.
After split testing multiple strategies, we moved from manual CPC bidding to tROAS bidding, leveraging machine learning algorithms to adjust bids and allocate budgets effectively. The use of tROAS bid strategies provided significant improvements in ad performance, enhancing the return on ad spend and contributing to the client's remarkable revenue growth. Adding more signals provides Google with more data to learn from, driving better performance. (Quick tip - Be sure that your feedback loop is closed: Ensure your conversions have a value associated with each. This is crucial for tROAS.)
How You Can See Similar Results With Google Ads AI/ML Features
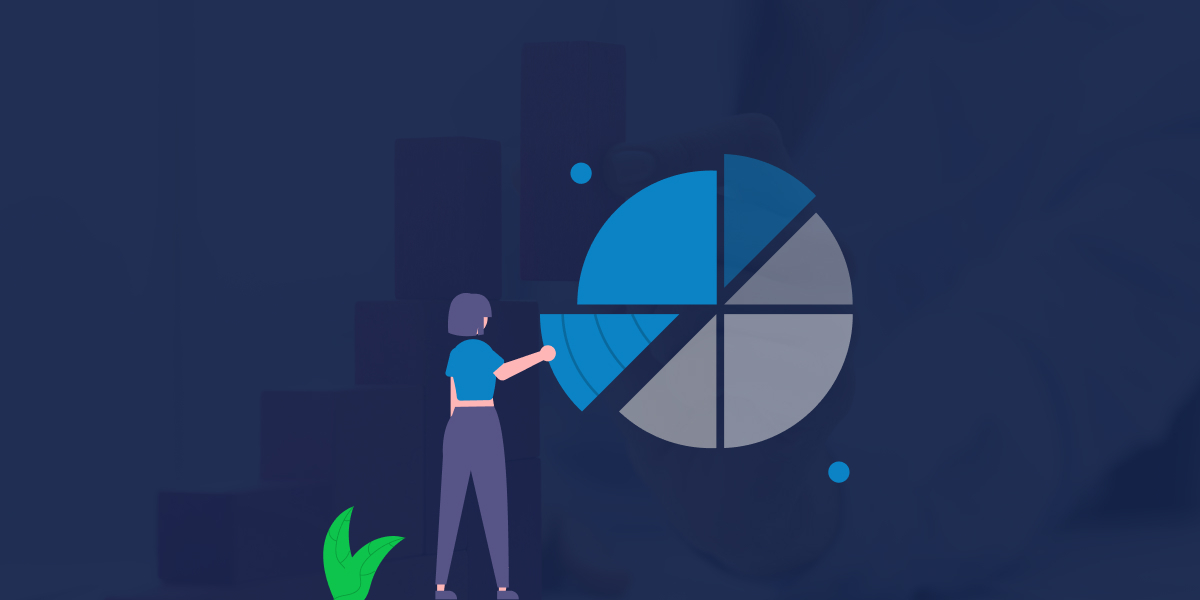
By reshaping our client's advertising setup, optimizing their data for Google's machine learning, and using strategies focused on getting the most value out of each ad dollar spent.
We were able to achieve an impressive boost in returns from their ad spend. By using our own unique data methods and the power of machine learning, we made their ads more effective, increased returns, and brought about a big jump in revenue.
If you're looking for Google Ads consultants to help boost your advertising campaigns, our track record proves we can. We're a professional group with unmatched expertise and constant support.
We're also easy to work with ;)
Our goal is to help you fully use the benefits of machine learning in advertising and unlock the huge potential for growth in your marketing efforts. After all, we do have a full blown analytics consulting team that allows us to help nearly any type of business collect, organize, and activate their customer data to drive growth and productivity with AI & ML.