Our Enterprise Architects & Data Engineers Can Help You
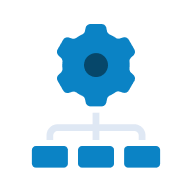
Google Cloud Platform Setup
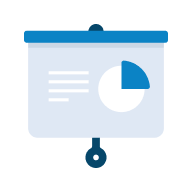
Setup Google Data Sources
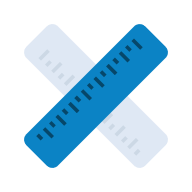
Design Your Data Warehouse
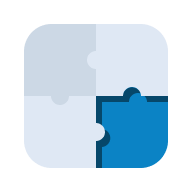
Extract Transform Load (ETL)
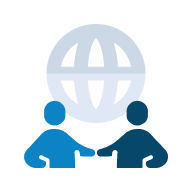
Integrate Your 3rd Party Data
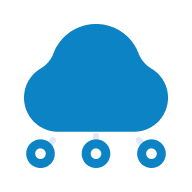
Migrate From
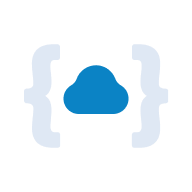
Move Data In GCP
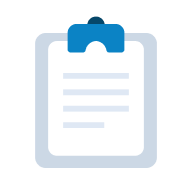
Migration Process
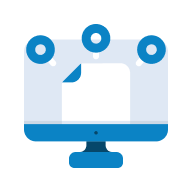
Advanced Analytics and ML
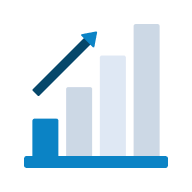
Real-Time Reporting, Dashboards & Alerts
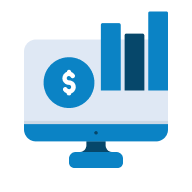
Query Optimization
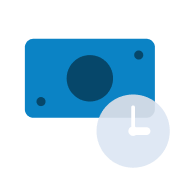
Cost Management and Budgeting
Our Enterprise Architects & Data Engineers Can Help You
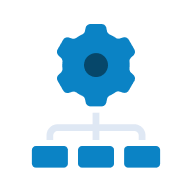
Google Cloud Platform Setup
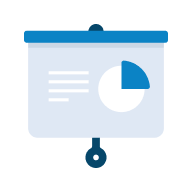
Setup Google Data Sources
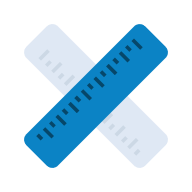
Design Your Data Warehouse
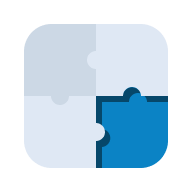
Extract Transform Load (ETL)
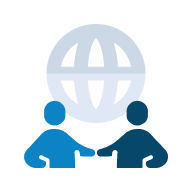
Integrate Your 3rd Party Data
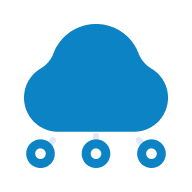
Migrate From
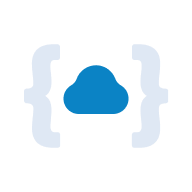
Move Data In GCP
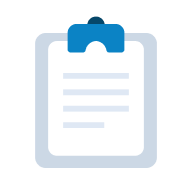
Migration Process
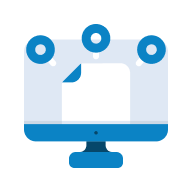
Advanced Analytics and ML
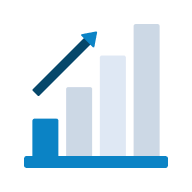
Real-Time Reporting, Dashboards & Alerts
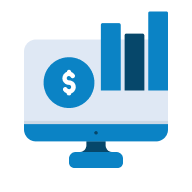
Query Optimization
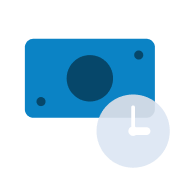
Cost Management and Budgeting
"The Three Ventures team led a project to migrate our existing Universal Analytics site into Google Analytics 4. The goal of the project was to achieve parity with our existing analytics program, including the migration and set-up of our Google Tag Manager accounts, as well as build reporting dashboards in Tableau using Big Query that would mirror the existing Data Studio reporting we had been using."
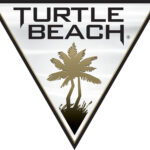
Enterprise Architects & Engineers For BigQuery Are Only A Click Away
BigQuery FAQ
BigQuery has a freemium model of pricing. All users get 10 gigabytes of storage and one terabyte of queries per month free of charge. Keep in mind that to use your data, you have to store it. Therefore, there are two types of costs associated with BigQuery. The first is storage – or how much data you will have to run queries against.
The second is analysis pricing, or paying for the computational resources to run a query against your data.
Pricing can be on a “pay as you go” model or a reserve model. Pay as you go is more expensive, but you only pay for what is used.
A reserve model is like signing a contract. You guarantee a price that you will pay per month, with a dollar amount minimum. You’ll get better pricing, but you’re on the hook for a minimum fee.
For current rates, please see BigQuery pricing or contact Three Ventures for support.
BigQuery integrates seamlessly with various data sources including Google Cloud Storage, transactional databases via federated data sources, and streaming data for real-time analysis. Tools like Cloud Dataflow and Cloud Dataprep can also be used to facilitate data integration and preprocessing.
BigQuery provides robust security features like data encryption at rest and in transit, Identity and Access Management (IAM) for fine-grained access control, and compliance with standards such as GDPR and HIPAA.
BigQuery is ideal for analytical workloads on large datasets, including data warehousing, ad-hoc queries, and business intelligence applications. It also supports real-time analytics through its streaming data capabilities.
Data can be migrated to BigQuery through batch loading from various data sources, streaming data in real-time, or using Google's Data Transfer Service for automated data ingestion from SaaS applications.
BigQuery ML allows users to create and execute machine learning models directly in the database using familiar SQL queries, enabling predictive analytics and data-driven decision-making without moving data.
To optimize BigQuery queries, use partitioned tables, employ clustering, select only necessary fields, use approximate aggregates when precision is not critical, and avoid anti-patterns like repeatedly transforming data via SQL functions.
BigQuery works with Google Cloud Dataflow for processing data pipelines and Cloud Composer, a managed Apache Airflow service, to orchestrate these workflows, ensuring efficient data handling and pipeline reliability.
BigQuery supports CDC by integrating with tools like Datastream, which captures and replicates data changes from source databases to BigQuery, enabling real-time analytics and historical data tracking.
BigQuery allows schema modifications on the fly without downtime, supports schema auto-detection during data loads, and encourages designing flexible schemas that can evolve with business needs.
Best practices include using Cloud Composer to manage and monitor workflow orchestration, setting up error handling and retry mechanisms, and ensuring that data dependencies are clearly defined and managed throughout the data pipeline.
Strategies include using version-controlled datasets, implementing views to abstract underlying schema changes, and utilizing features like schema evolution for modifying table schemas without disrupting existing queries.
Enterprise Architects & Engineers For BigQuery
Thank you for getting in touch!
We will get back to you as soon as possible
(usually within one work day).